Profile for Chad Vidden
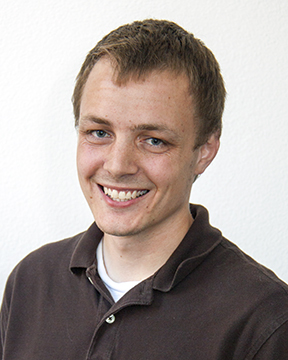
Contact me
Chad Vidden
Professor
Mathematics & Statistics
University of Wisconsin-La Crosse
Chad Vidden
Professor
Mathematics & Statistics
Specialty area(s)
- Intersections of mathematics and AI / big data
- Machine learning, AI, and data science with applications in various fields of study
- Applied mathematics, numerical analysis, and computational mathematics
- Undergraduate research
Brief biography
I am currently a Professor of Mathematics at UWL where my interests include:
- teaching undergraduate mathematics with special emphasis on applications,
- collaborating with student researchers on data driven projects,
- the leading edge of machine learning and AI and mathematics involved,
- and collaborating with local companies on research and student opportunities.
Education
Ph.D. Applied Mathematics, Iowa State University, 2013
B.S. Mathematics, Minnesota State University - Mankato, 2007
Career
Teaching history
MTH 150, 151, 207, 208, 308, 309, 310, 353, 371, 480, 498, STAT 145
Undergraduate Research Projects:
- Numerical Methods for Differential Equations
- Sports Ranking through Linear Algebra
- Machine Learning
- Exoplanet Detection through Fast Fourier Transform
Professional history
Professor of Mathematics, University of Wisconsin - La Crosse, July 2022 - current.
Associate Professor of Mathematics, University of Wisconsin - La Crosse, July 2018 - June 2022.
Assistant Professor of Mathematics, University of Wisconsin - La Crosse, August 2013 - June 2018.
Assistant Professor of Mathematics, University of Wisconsin - Platteville, August 2012 - May 2013.
Graduate Teaching Assistant, Iowa State University, August 2007 - May 2012.
Teaching Assistant, Minnesota State University - Mankato, August 2005 - May 2006.
Research and publishing
Textbook publication:
- From Data to Decision: A Handbook for the Modern Business Analyst by M. Vriens, S. Chen, and C. Vidden, Cognella Academic Publishing, December 2018. (second edition 2023)
Machine learning, data science, business, education:
- S. Floersch, C. Vidden, A. Jagim, et al. "Seasonal Changes in Match Demands and Workload Distribution in Collegiate Soccer Across Two Seasons". Journal of Strength and Conditioning Research, 2024.
- M. Vriens, N. Bosch, C. Vidden, and J. Talwar. "Prediction and profitability in market segmentation typing tools". Journal of Marketing Analytics, 2022.
- M. Vriens, C. Vidden, and N. Bosch. "The benefits of Shapley-value in key-driver analysis." Applied Marketing Analytics, 2021.
- M. Vriens, C. Vidden, and J. Schomaker. "What I see is what I want: Top-down attention biasing choice behavior," Journal of Business Research, 2020.
- M. Vriens and C. Vidden. "The Linux Compete strategy: An analytics case study." Applied Marketing Analytics, 2019.
- M. Vriens, S. Chen, and C. Vidden. "Mapping brand similarities: Comparing consumer online comments versus survey data." International Journal of Market Research, 2019.
- S. Chen, C. Vidden, N. Nelson, and M. Vriens. "Topic modelling for open-ended survey responses." Applied Marketing Analytics, 2018.
- M. Vriens, C. Vidden, S. Chen, and S. Kaulartz. "Assessing the impact of a brand crisis using big data: The case of the VW Diesel emission Crisis." DMA Annual Analytics Journal, 2017.
- C. Vidden, M. Vriens, and S. Chen. "Comparing clustering methods for market segmentation: A simulation study." Applied Marketing Analytics, 2016.
Numerical analysis, discontinuous Galerkin methods, applied mathematics:
- C. Vidden. "A new approach for admissibility analysis of the direct discontinuous Galerkin method through Hilbert matrices." Numer. Methods Partial Differential Equations, 2016.
- C. Vidden and J. Yan. "A new direct discontinuous Galerkin method with symmetric structure for nonlinear diffusion equations." Journal of Computational Mathematics, 2013.
- Invariant measures for hybrid stochastic systems (with X. Garcia, J. Kunze, T. Rudelius, A. Sanchez, S. Shao, E. Speranza). Involve, 2014.
- Trading cookies in a gambler's ruin scenario (with K. Jungjaturapit, T. Pluta, R. Rastegar, A. Roiterschtein, M. Temba, B. Wu). Involve, 2013.
Undergraduate research (mentored):
- S. Floersch. "Why the 2020 Dodgers are the Greatest Team of all Time, at Least Statistically." UWL Journal of Undergraduate Research, 2021.
- Q. Burzynski, L. Frank, Z. Nordstrom, J. Wolfe. "Profit Optimization." UWL Journal of Undergraduate Research, 2018. (joint with S. Chen)
- J. Meyers, D. Morrison. "Math Department Schedule Optimization." UWL Journal of Undergraduate Research, 2016. (joint with S. Chen)
Kudos
published
published
published